Generative ML in chemistry is bottlenecked by synthesis
4.4k words, 21 minutes reading time
Note: I am not a chemistry expert. Huge shout-out to Anand Muthuswamy, Gabriel Levine, and Corin Wagen for their incredible help in correcting my misunderstandings here! But some may remain, please DM me or comment if you see one!
Also, PostEra is a sponsor on this post! They are one of the few small-molecule ML startups that specialize in helping address synthesizability challenges in generative models. This is distinct from the ‘synthesis problem’ I’ll focus on in this essay, but, as we’ll see, addressing synthesizability in generative models can help alleviate synthesis issues.
For transparency's sake: outside of a request to add in two PostEra-produced works (Manifold and a paper), both of which were highly relevant, PostEra had no other input on this piece.
Introduction
Every single time I design a protein — using ML or otherwise — I am confident that it is capable of being manufactured. I simply reach out to Twist Biosciences, have them create a plasmid that encodes for the amino acids that make up my proteins, push that plasmid into a cell, and the cell will pump out the protein I created.
Maybe the cell cannot efficiently create the protein. Maybe the protein sucks. Maybe it will fold in weird ways, isn’t thermostable, or has some other undesirable characteristic.
But the way the protein is created is simple, close-ended, cheap, and almost always possible to do.
The same is not true of the rest of chemistry. For now, let’s focus purely on small molecules, but this thesis applies even more-so across all of chemistry.
Of the 1060 small molecules that are theorized to exist, most are likely extremely challenging to create. Cellular machinery to create arbitrary small molecules doesn’t exist like it does for proteins, which are limited by the 20 amino-acid alphabet. While it is fully within the grasp of a team to create millions of de novo proteins, the same is not true for de novo molecules in general (de novo means ‘designed from scratch’). Each chemical, for the most part, must go through its custom design process.
Because of this gap in ‘ability-to-scale’ for all of non-protein chemistry, generative models in chemistry are fundamentally bottlenecked by synthesis.
This essay will discuss this more in-depth, starting from the ground up of the basics behind small molecules, why synthesis is hard, how the ‘hardness’ applies to ML, and two potential fixes. As is usually the case in my Argument posts, I’ll also offer a steelman to this whole essay.
To be clear, this essay will not present a fundamentally new idea. If anything, it’s such an obvious point that I’d imagine nothing I’ll write here will be new or interesting to people in the field. But I still think it’s worth sketching out the argument for those who aren’t familiar with it.
What is a small molecule anyway?
Typically organic compounds with a molecular weight under 900 daltons. While proteins are simply long chains composed of one-of-20 amino acids, small molecules display a higher degree of complexity. Unlike amino acids, which are limited to carbon, hydrogen, nitrogen, and oxygen (and sometimes sulfur), small molecules incorporate a much wider range of elements from across the periodic table. Fluorine, phosphorus, bromine, iodine, boron, and chlorine have all found their way into FDA-approved drugs.
This elemental variety gives small molecules more chemical flexibility but also makes their design and synthesis more complex. Again, while proteins benefit from a universal ‘protein synthesizer’ in the form of a ribosome, there is no such parallel amongst small molecules! People are certainly trying to make one, but there seems to be little progress.
So, how is synthesis done in practice?
For now, every atom, bond, and element of a small molecule must be carefully orchestrated through a grossly complicated, trial-and-error reaction process which often has dozens of separate steps. The whole process usually also requires non-chemical parameters, such as adjusting the pH, temperature, and pressure of the surrounding medium in which the intermediate steps are done. And, finally, the process must also be efficient; the synthesis processes must not only achieve the final desired end-product, but must also do so in a way that minimizes cost, time, and required sources.
How hard is that to do? Historically, very hard.
Consider erythromycin A, a common antibiotic.
Erythromycin was isolated in 1949, a natural metabolic byproduct of Streptomyces erythreus, a soil microbe. Its antimicrobial utility was immediately noticed by Eli Lilly; it was commercialized in 1952 and patented by 1953. For a few decades, it was created by fermenting large batches of Streptomyces erythreus and purifying out the secreted compound to package into therapeutics.
By 1973, work had begun to artificially synthesize the compound from scratch.
It took until 1981 for the synthesis effort to finish. 9 years, focused on a single molecule.
Small molecules are hard to fabricate from scratch. Of course, things are likely a lot better today. The space of known reactions has been more deeply mapped out, and our ability to predict reaction pathways has improved dramatically. But arbitrary molecule creation at scale is fully beyond us; each molecule still must go through a somewhat bespoke synthesis process.
Okay, but…why? Why is synthesis so hard?
Why is synthesis hard?
Remember those ball-and-chain chemistry toys you used as a kid? Let’s say you have one in your hands. Specifically, something referred to as a ‘benzene ring’. The black balls are carbon, and the white balls are hydrogen. The lines that connect it all are chemical bonds; one means a single bond, and two means a double bond.
Now let’s say you want to alter it. Maybe you want to add on a new element. Let’s also say that the toy now suddenly stays to obey the actual atomic laws that govern its structure. You pluck out an atom, a hydrogen from the outer ring, so you can stick on another element. The entire structure dissolves in your hands as the interatomic forces are suddenly unevenly balanced, and the atoms are ripped away from one another.
Oops.
Do you know what you actually should’ve done? Dunk the benzene into a dilute acid and then add in your chemical of interest, which also has to be an electrophile. In chemistry terms, perform an electrophilic aromatic substitution — a common way to modify benzene rings. The acid creates an environment where near-instantaneous substitution of the hydrogen atom can occur, without it destabilizing the rest of the ring.
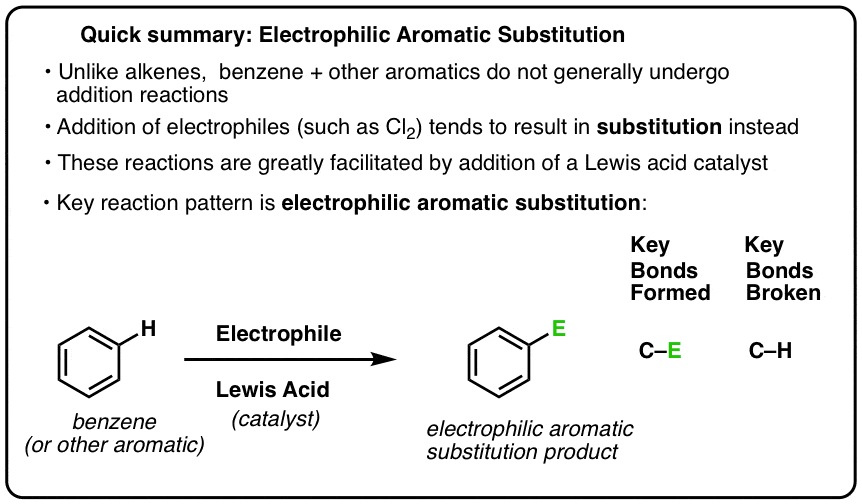
How were you supposed to know that?
In practice, you’d have access to retrosynthesis software like SYNTHIA or MANIFOLD or just dense organic chemistry synthesis textbooks to figure this out. But, also in practice, many reaction pathways literally don’t exist or are challenging to pull off. There’s a wonderful 2019 essay that is illustrative of this phenomenon: A wish list for organic chemistry1. The author points out five reaction types (fluorination, heteroatom alkylation, carbon coupling, heterocycle modification, and atom swapping) that are theoretically possible, would be extremely desirable to have in a lab, but have no practical way of being done. Of course, there is a way to ‘hopscotch around’ to some desirable end-product using known reactions, but that has its own problems we’ll get to in a second.
Let’s try again. You’re given another toy benzene ring. Actually, many millions of benzene rings, since you’d like to ensure your method works in bulk.
You correctly perform the electrophilic aromatic substitution using your tub of acid, replacing the hydrogen with an electrophile. Well, that’s nice, you've got your newly modified benzene floating in a sea of acid. But you look a bit closer. Some of our benzene rings got a little too excited and decided to add two substituents instead of one, or broke apart entirely, leaving you with a chemical soup of benzene fragments. You also have a bunch of acid that is now useless.
This felt…inefficient.
Despite using the ostensibly correct reaction and getting the product we wanted, we now have a ton of waste, including both the acid and reactant byproducts. It’s going to take time and money to deal with this! In other words, what we just did had terrible process mass intensity (PMI). PMI is a measure of how efficiently a reaction uses its ingredients to achieve the final end-product. Ideally, we’d like the PMI to be perfect, every atom involved in the reaction process is converted to something useful. But some reaction pathways have impossible-to-avoid bad PMI. Unfortunately, our substitution reaction happened to be one of those.
Okay, fine, whatever, we’ll pay for the disposal of the byproducts. At least we got the modified benzene. Given how much acid and benzene we used, we check our notes and expect about 100 grams of the modified benzene to have been made. Wonderful! Now we just need to extract the modified benzene from the acid soup using some solvents and basic chemistry tricks. We do exactly that and weigh it just to confirm we didn’t mess anything up. We see 60 grams staring back at us from the scale.
What? What happened to the rest?
Honestly, any number of things. Some of the benzene, as mentioned, likely just didn’t successfully go through with the reaction, either fragmenting or becoming modified differently. Some of the benzene probably stuck to our glassware or got left behind in the aqueous layer during extraction. A bit might have evaporated, given how volatile benzene and its derivatives can be. Each wash, each transfer between containers, and each filtration step all took its toll. The yield of the reaction wasn’t perfect and it basically never is.
The demons of PMI, yield, and other phenomena is why the ‘hopscotching around’ in reactions is a problem! Even if we can technically reach most of chemical space through many individual reactions, the cost of dealing with byproducts of all the intermediate steps and the inevitably lowered yield can be a barrier to exploring any of it. Like, let’s say you've got a 10-step synthesis planned out, and each step has a 90% yield (which would be extremely impressive in real life). The math shakes out to be the following: 0.9^10 = 0.35 or 35%. You're losing 65% of your theoretical product from yield losses.
Moreover, our benzene alteration reaction was incredibly simple. A ten-step reaction — even if well-characterized — will likely involve trial and error, thousands of dollars in raw material, and a PhD-level organic chemist working full-time at 2 steps/day. And that’s if the conditions of each reaction step is already well-established! If not, single steps could take weeks! All for a single molecule.
Synthesis is hard.
How the synthesis bottleneck manifests in ML
The vast majority of the time, generative chemistry papers will assess themselves in one of three ways:
Purely in-silico agreement using some pre-established reference dataset. Here is an example.
Using the model as a way to do in-silico screening of a pre-made chemical screening library (billions of molecules that are easy to combinatorially create at scale), ordering some top-N subset of the library, and then doing in-vitro assessment. Here is an example.
Using the model to directly design chemicals de novo, synthesizing them, and then doing in-vitro assessment. Here is an example.
1 is insufficient to really understand whether the model is useful. Unfortunately, it also makes up the majority of the small-molecule ML papers out there, since avoiding dealing with the world of atoms is always preferable (and something I sympathize with!).
2 is a good step — you have real-world data — but it still feels limited, does it not? Because you’re relying on this chemical screening library, you’ve restricted your model to the chemical space that is easily reachable through a few well-understood reactions and building blocks. This may not be a big deal, but ideally, you don’t want to restrict your model at all, even if this method allows you to scale synthesis throughput.
3 should be the gold standard here, you’re generating real data, but also giving full creative range to the generative model. But, if you look at the datasets associated with 3, you’ll quickly notice a big issue: there’s an astonishingly low number of molecules that are actually synthesized. It is consistently in the realm of <25 generated molecules per paper! And it’s often far, far below that. We shouldn’t be surprised by this, given how many words of this essay have been dedicated to emphasizing how challenging synthesis is, but it is still surprising to observe.
In terms of anecdotes, I put up this question on my Twitter. The replies generally speak for themselves — basically no one disagreed, placing the blame on synthesis (or a lack of wet-lab collaborations) for why such little real-world validation exists for these models.
Returning to the thesis of this post, what is the impact of all of this synthesis difficulty on generative models? I think a clean separation would be model creativity, bias, and slow feedback loops. Let’s go through all three:
Model creativity. While generative models are theoretically capable of exploring vast swathes of chemical space, the practical limitations of synthesis force their operators to use a narrow slice of the model’s outputs. Typically, this will mean either ‘many easily synthesizable molecules’ or ‘a few hard-to-synthesize molecules’. But, fairly, while restraining the utility of a model’s outputs may immediately seem bad, it’s an open question how much this matters! Perhaps we live in a universe where ‘easily synthesizable’ chemical space matches up quite well with the space of ‘useful’ chemical space? We’ll discuss this a bit more in the Steelman section, but I don’t think anyone has a great answer for this.
Bias. Now, certainly, some molecules can be synthesized. And through chemical screening libraries, which allow us a loophole out from this synthesizability hell by combinatorially creating millions of easily synthesizable molecules, we can create even more molecules! Yet, it seems like even this data isn’t enough, many modern ‘representative’ small molecule datasets are missing large swathes of chemical space. Furthermore, as Leash Bio pointed out with their BELKA study, models are really bad at generalizing beyond the immediate chemical space they were trained on! Because of this, and how hard it is to gather data from all of chemical space, the synthesis problem reigns largest here.
Slow feedback loops. As I’ve discussed at length in this essay, synthesis is hard. Really hard. I mentioned the erythromycin A synthesis challenge earlier, but even that isn’t the most egregious example! The anticancer drug Paclitaxel took decades to perform a total synthesis of, extending into the early 2020’s! Fairly, both of these fall into the corner of ‘crazy natural products with particularly challenging synthesis routes’. But still, even routine singular chemicals can take weeks to months to synthesize. This means that upon discovering strong deficiencies in a generative chemistry model, providing the data to fix that deficiency can take an incredibly long of time.
As a matter of comparison, it’s worth noting that none of three problems are really the case for proteins! Companies like Dyno Therapeutics (self-promotion) and A-Alpha Bio (who I have written about before) can not only computationally generate 100k+ de novo proteins, but also physically create and assess them in the real world, all within a reasonably short timeframe. Past that, proteins may just be easier to model. After all, while protein space is also quite large, there does seem to be a higher degree of generalization in models trained on them; for example, models trained on natural protein data can generalize beyond natural proteins.
Small molecules have a rough hand here: harder to model and harder to generate data for. It is unlikely the bitter lesson cannot be applied here; the latter problem will be necessary to solve to fix the former.
Potential fixes
Synthesis-aware generative chemistry
Let’s take a step back.
Synthesizability has been discussed in the context of generative chemistry before, but the discussion usually goes in a different direction. In a 2020 paper titled ‘The Synthesizability of Molecules Proposed by Generative Models’, the authors called out an interesting failure mode of generative chemistry models. Specifically, they often generate molecules that are physically impossible or highly unstable. For instance, consider some ML-generated chemicals below:
Some molecules here look fine enough. But most here look very strange, with an impossible number of bonds attached to some elements or unstable configurations that would break apart instantly. This is a consistently observed phenomenon in ML-generated molecules: okay at a glance, but gibberish upon closer inspection. We’ll refer to this as the ‘synthesizability problem’, distinct from the ‘synthesis problem’ we’ve discussed so far.
Now, upon first glance, the synthesizability problem is interesting, but it’s unclear how much it really matters. If you solve the synthesizability issue in generative models tomorrow, how much value have you unlocked? If your immediate answer is ‘a lot’, keep in mind that solving the synthesizability issue really only means you can successfully constrain your model to only generate stable things that are physically plausible. As in, molecules that don’t immediately melt and molecules that don’t defy the laws of physics. This is some constraint on the space of all chemicals, but it feels enormously minor.
Of course, improving overall synthesis capabilities is far more important. But how realistic is that? After all, achieving broadly easier synthesis is the holy grail of organic chemistry.
It feels like each time someone successfully chips away at the problem, they are handed a Nobel Prize (e.g. Click Chemistry)! And it’d make for a boring and, to chemists, aggravating essay if our conclusion was ‘I recommend that the hardest known problem in the field should be solved’. Let’s be more realistic.
How do we make the most of the models we already have?
Let’s reconsider the synthesizability problem. If we cannot realistically scale up the synthesis of arbitrary molecules, we could at least ensure that the generative models we’re working with will, at least, give us synthesizable molecules. But we should make a stronger demand here: we want not just molecules that are just synthetically accessible, but molecules that are simple to synthesize.
What does that mean? One definition could be low-step reaction pathways that require relatively few + commercially available reagents, have excellent PMI, and have good yield. Alongside this, we’d also like to know the full reaction pathway too, along with the ideal conditions of the reaction! It’s important to separate out this last point from the former; while reaction pathways are usually immediately obvious to a chemist, fine-tuning the conditions of the reactions can take weeks.
Historically, at least within the last few years, the ‘synthesizability problem’ has been only directed towards chemical accessibility. The primary way this was done was by not using chemicals as training data, but only incorporating retrosynthesis-software-generated reaction pathways used to get there. As such, at inference time, the generative model does not propose a single molecule, but rather a reaction pathway that leads to that molecule. While this ensures synthetic accessibility of any generated molecule, it still doesn’t ensure that the manner of accessibility is at all desirable to a chemist who needs to carry out hundreds of these reactions. This is partially because ‘desirability’ is a nebulous concept. A paper by MIT professor Connor Coley states this:
One fundamental challenge of multi-step planning is with the evaluation of proposed pathways. Assessing whether a synthetic route is “good” is highly subjective even for expert chemists….Human evaluation with double-blind comparison between proposed and reported routes can be valuable, but is laborious and not scalable to large numbers of pathways
Moreover, while reaction pathways for generated molecules are decent, even if the problem of desirability is still being worked out, predicting the ideal conditions of these reactions is still an unsolved problem. Derek Lowe wrote about a failed attempt to do exactly this in 2022, the primary problem being that historical reaction pathway datasets are untrustworthy or have confounding variables.
But there is reason to think improvements are coming!
Derek covered another paper in 2024, which found positive results in reaction condition optimization using ML. Here, instead of relying purely on historical data, their model was also trained using a bandit optimization approach, allowing it to learn in the loop, balancing between the exploration of new conditions and the exploitation of promising conditions. Still though, there are always limitations with these sorts of things, the paper is very much proof-of-concept. Derek writes:
The authors note that even the (pretty low) coverage of reaction space needed by this technique becomes prohibitive for reactions with thousands of possibilities (and those sure do exist), and in those cases you need experienced humans to cut the problem down to size.
In a similar vein, I found another interesting 2024 paper that discusses ‘cost-aware molecular design’. This isn’t an ML paper specifically, but instead introduces an optimization framework for deciding between many possible reaction pathways used for de novo molecules. Importantly, the framework considers batch effects in synthesis. It recognizes that making multiple related molecules can be more efficient than synthesizing each one individually, as they may share common intermediates or reaction steps. In turn, this allows it to scale up to hundreds of candidate molecules.
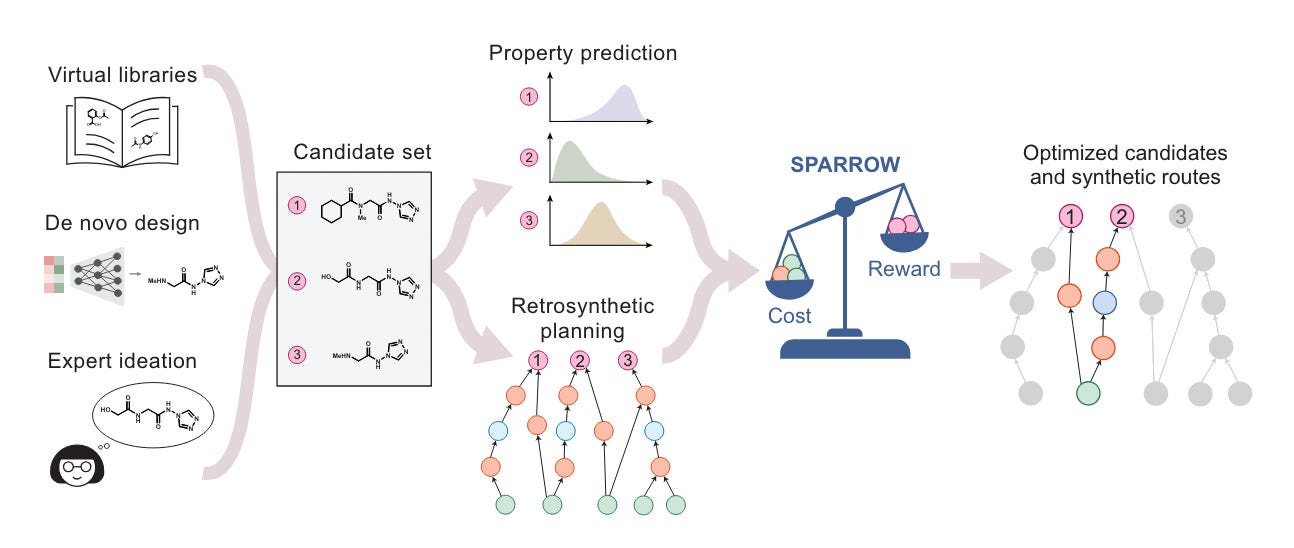
Of course, there is still lots of work left to do to incorporate these frameworks into actual generative models and to fully figure out reaction condition optimization. Furthermore, time will tell how much stuff like this makes a difference versus raw improvements in synthesis itself.
But in the short term, I fully expect computational advancements in generated molecule synthesizability, synthesis optimization, and synthesis planning to deliver an immense amount of value.
Improvements in synthesis
Despite the difficulty of it, we could dream a little about potential vast improvements in our ability to synthesize things well. Unlike with ML, there is unfortunately no grand unification of arbitrary synthesis theories about, it’s more in the realm of methods that can massively simplify certain operations, but not others.
One of the clearest examples here is skeletal editing, which is a synthesis technique pioneered in just the last few years. Most reactions can only operate on the ‘edges’ of a given chemical, removing, swapping, or adding molecules from the peripheries. If you want to pop out an atom from the core of a molecule and replace it with something else, you’ll likely need to start your chemical synthesis process from scratch. But the advent of skeletal editing has changed this! For some elements in some contexts, you are able perform a precise swap. Switching a single internal carbon to a single [other thing] in a single reaction, and so on. As always, Derek Lowe has previously commented on the promise of this approach.
Here is a review paper that discusses skeletal editing in more detail, alongside other modern methods that allow for easier synthesis. All of them are very interesting, and it would be unsurprising for some of them to be serious contenders for a Nobel Prize. It may very well be the case that we’ve only scratched the surface of what’s possible here, and further improvements in these easier synthesis methods will, by themselves, allow us to collect magnitudes more data than previously possible.
But for now, while techniques like skeletal editing are incredible, they are really only useful for late-stage molecule optimization, not for the early stages of lead finding. What of this grand unification of chemical synthesis we mentioned earlier? Is such a thing on the horizon? Is it possible there is a world in which atoms can be precisely stapled together in a singular step using nanoscale-level forces? A ribosome, but for chemicals in general?
Unfortunately. this probably isn’t happening anytime soon. But, as with everything in this field, things could change overnight.
Steelman
A steelman is an attempt to provide a strong counter-argument — a weak one being a strawman — to your own argument. And there’s a decent counterargument to this whole essay! Maybe not exactly a counterargument, but something to mentally chew on.
In some very real sense, the synthesis of accessible chemical space is already solved by the creation of virtual chemical screening libraries. Importantly, these are different from true chemical screening libraries, in that they have not yet been made, but are hypothesized to be pretty easy to create using the same combinatorial chemistry techniques. Upon ordering a molecule from these libraries, the company in charge of it will attempt synthesis and let you know in a few weeks if it's possible + send it over if it is.
One example is Enamine REAL, which contains 40 billion compounds. And as a 2023 paper discusses, these ultra-large virtual libraries display a fairly high number of desirable properties. Specifically, dissimilarity to biolike compounds (implying a high level of diversity), high binding affinities to targets, and success in computational docking, all while still having plenty of room to expand.
Of course, it is unarguable that 40 billion compounds, large as it is, is a drop in the bucket compared to the full space of possible chemicals. But how much does that matter, at least for the moment? Well, we do know that there are systemic structural differences between combinatorially-produced chemicals and natural products (which, as discussed with erythromycin A and paclitaxel, are challenging to synthesize). And, historically, natural products are excellent starting points for drug discovery endeavors.
But improvements in life-science techniques can take decades to trickle down to functional impacts, and the advent of these ultra-massive libraries are quite a bit younger than that! Perhaps, over the next few years — as these virtual libraries are scaled larger and larger — the problems that synthesis creates for generative chemistry models are largely solved. Even if structural limitations for these libraries continue to exist, that may not matter, data will be so plentiful that generalization will occur.
For now, easy de novo design is valuable, especially given that the compounds contained in Enamine REAL are rarely the drugs that enter clinical trials. But, perhaps someday, this won’t be the case, and the set of virtual screening space will end up encompassing all practically useful chemical space.
That’s all, thank you for reading!
If curious, in 2023, Corin Wagen wrote up a post analyzing how the field has shifted in the 4 years since the ‘wish list’ was published. Lots of progress on two of the five, little on the rest.
Maybe this is dumb but:
manufacturing costs are a trivial fraction of the cost of bringing a drug to market. So are drug discovery costs. So why should we completely dismiss the possibility of a startup that spends way more on building libraries of chemicals with "inefficient" syntheses, in the hopes that the Cool AI will discover some new small molecules? If any are successful, the higher manufacturing costs will be peanuts next to the revenue associated with a whole new drug class!
Very nice article.
I would add that synthesis is only one of the lab-based bottlenecks for generative ML models, because we don't merely want our model to propose synthesizable compounds. We want it to propose useful ones.
I.e., we want molecules that hit the target, don't have undesirable off-target effects, are sufficiently soluble, have good intestinal absorption, have good liver clearance, are nontoxic, etc. To achieve this computationally with ML, we need sufficiently large data sets for each of these attributes to train good models. And the models need to give good predictions for the region of chemical space relevant to our particular project, so a liver clearance model trained using data from the project down the hall might not be applicable to our own. A major bottleneck to creating the needed data sets is the speed at which compounds can be assayed in the lab.