Why Recursion Pharmaceuticals abandoned cell painting for brightfield imaging
5.7k words, 26 minutes reading time
Note: thank you to Brita Belli, senior communications manager at Recursion, for connecting me to Charles Baker, a VP at Recursion, who led a lot of this work I’ll discuss here + allowed me to interview him about it! I am not affiliated with Recursion in any capacity.
One more note: a few people have pointed it out that I got the cell painting invention date wrong. It’s actually 2013 from this paper, and not 2016 from this paper. My bad! A few words here have been updated to account for this.
Introduction
At this point, you’d be hard pressed to not have heard of Recursion Pharmaceuticals.
They were one of the first biotechs to apply machine-learning (ML) to the problem of drug discovery back in 2013 and, currently, seem to be the only winners in the area. I use ‘winner’ loosely of course — they have yet to push a drug to market and their recent Phase 2 readouts were disappointing (here is some nuance on the whole subject that most article leave out though1). Of course, caveats on this readout (maybe) being an unfairly negative assessment of the company’s capabilities, given that it was Chris Gibson’s — the CEO of Recursion — PhD project from 20132, and drugs take time to develop.
Nevertheless, they have done something that very few others in their shoes have: survive for years and not be under active threat of going under.
This is no small feat in a field filled with otherwise promising companies that have failed that deceptively simple smell test, Atomwise being the latest case. I am as unsure as anyone as to whether Recursion’s more grandiose promises, (discussed more in detail by Derek Lowe here) will ever come to pass, but at least they are still around.
The bet of the whole company is the following: take a drug (either from a chemical screening library or dreamt up by a model), wash it over a plate of cells, visually observe how the cells react to it, and repeat this a few billion times across many different cells + many genetic knockouts of those cells (which acts as a model of a disease) + many different drugs. The whole idea is also known as phenomics — the study of the visual phenotype of organisms. In this case, the ‘organism’ is a small set of cells. Once you’ve done that, train a model on those petabytes of cellular images, along with the genetic or chemical perturbation applied.
The Recursion bet is that the resulting model would acquire an extremely strong understanding of the interaction between the visual morphology of cells, their genetic makeup, and drugs applied to them — predicting the rest given one (or two) of the others. From there on out, you can do any number of things:
Screen new drugs by comparing the image of cells given that drug to images of cells with genetic alterations that model a given disease.
Identify novel mechanisms of disease by looking at how the phenomic clusters of gene alterations cluster with phenomic signals from genes known to be associated with certain diseases.
Study cell morphology relationships by clustering large sets of genetically perturbed samples.
And so on.
It’s a fun idea! You can compare this phenotype-based approach to something like target-based discovery, where you have a cellular target in mind (insulin receptor, adrenergic receptor, etc) and want to optimize a therapeutic to do [something] to it. Historically, target-based discovery has a pretty bad success rate, phenotype-based approaches do much better (though, as with everything, it’s nuanced). This shouldn’t be too surprising; biology is complex, and dissolving things down to a single target is hard. The Recursion bet is not just on the phenotype-based approach, but also in scaling it up to an insane degree: >19 petabytes of cellular images + perturbation pairs at last count (2024).
Has this paid off?
Realistically, I think it’s still a work in progress. They have discussed the capabilities of such a model trained on such data (self-supervised, encoder-only transformer) in a paper presented at CVPR 2024, where it won a spotlight. The results are pretty interesting in vibe-space alone: a 75% masked image of cells, when put through their model, could yield a reasonably true-to-life filled-in image.
In terms of actual clinical utility, I think it’s a bit hard to say. While they do quite well on the benchmarks given in the paper, I think it’s an open question how good the benchmarks actually are. Or, at least, how well they translate to the main problems in taking drugs to market — target selection and toxicology. Really though, opining on this all isn’t super useful for anyone; the final answer will be in what their clinical trial readouts will be. Of which there will be 6 more readouts in the next 18~ months per their 2024 Download Day in July (and 10 if counting their proposed merger with Exscientia). The one at the top, REC-994, wasn’t great, but perhaps the others stand a shot.
But this essay isn’t about Recursion’s drug discovery strategy. Much has already been written about that and I certainly don’t have a unique take there.
This essay is about how Recursion takes pictures of cells in the first place, why it (officially) changed its approach just a few months ago, and why I think the decision to change it is a lot more interesting than people think.
This post really stemmed from a LinkedIn post by Charles Baker, a VP at Recursion Pharmaceuticals, that I saw a few weeks ago. It described how the company had recently moved over from one cellular imaging modality (cell painting, created in 2013) to a much older one (brightfield imaging, arguably created in the 1500’s). Very, very important context: Recursion was founded on the former assay and had stuck to it for over a decade. Moving over to something new this late in the game was a surprising move!
Yet, relatively little has been written about it. There’s that one early post by Charles, one more by Brita Belli — a senior communications manager at Recursion — and that’s it.
This essay is meant to rectify the missing gap here.
What is brightfield imaging? What is cell painting? Why did Recursion focus on the latter first, and then switch to the former recently? And how did the transition process go internally? This essay will discuss all that.
What is brightfield imaging?
Brightfield imaging is, as mentioned, an old technique. Its origins can be traced back to the 15th century, when Dutch pioneers in optics — Hans and Zacharias Janssen — first invented the compound microscope. In the 17th century, Antonie Van Leeuwenhoek used improved versions of the microscope, capable of far greater magnifications, to observe microscopic life. Now, in the modern era, it is routinely used by scientists to study intricate cellular behavior.
At its start, brightfield imaging wasn’t even called so; it was simply the only way to observe things through a microscope. Only later was it named ‘brightfield’ to distinguish it from alternative, newer techniques (e.g. darkfield imaging). The principles of it are simple: you shine visible light through [something] and observe what comes out the other side. In the case of cells, you place them between glass slides, shine light from underneath them, and observe what's visible from the top using a microscope.
Different parts of a cell interact with light differently. The cell membrane, nucleus, and various organelles all have their own differences in light absorption, creating contrast in the final image. The result is a grayscale picture — as cells are generally colorless or transparent — where darker areas represent denser or more light-absorbing parts of the cell, while lighter areas show where light passes through more easily.
It's fundamentally equivalent to holding up a leaf to the sun — from the shadows arise structure. It’s incredibly cheap, simple, and easy to perform. As a further benefit, cells generally don’t care if light is being shone on them, so brightfield imaging doesn’t alter cell behavior either. For centuries, brightfield imaging was a way — again, the only way — for humans to deeply study the behavior of microscopic life, and it did that job splendidly.
There’s really only one real issue with brightfield imaging: it’s really, really hard to observe anything interesting. Here is a single cell, imaged using brightfield.
You can vaguely see some details about the state of the cell. Size, shape, and maybe some details about its internals. It’s hard to see much of anything of immediate note though — we’d really need to squint and stare at the fuzzy blob to get a sense of the structure.
In concrete terms, our problem here is one of ‘low contrast’.
We’re depending on the shadows of a cell to give us a sense of the structure, but it unfortunately seems like most of a cell is quite transparent. There is relatively little difference between the highest and lowest points of absorption across a cell. And, even more unfortunately, this problem of low contrast isn’t unique here, but a problem across the microscopic world. While a seasoned researcher who has only studied this one specific type of cell — say, hepatocytes — may not find this to be an issue when looking at hepatocytes, they may have trouble when looking at neurons. Cells have an immense level of heterogeneity, and brightfield imaging makes it quite hard for any one human to study many different types of cells without constant reference look-ups.
Is there a way we could improve the contrast of the cells? We could perhaps be a bit more clever on how light is shined through the biological specimen, as is done in phase-contrast microscopy. For example:
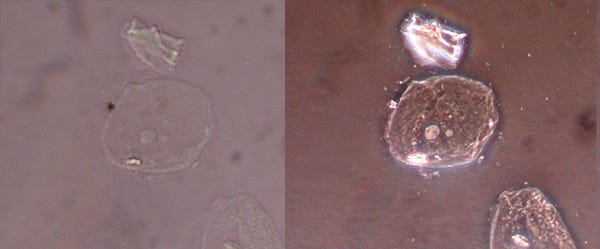
But the bump in contrast here is only partial, many of the finer details are missed or still yet obscured. Is there a better way? Yes: cell painting.
What is cell painting?
If you carefully sift through millions of chemicals, you’ll stumble across a set of dyes that are chemically attracted to specific cellular biomolecules. If these dyes are fluorescent — something that will absorb light and re-emit it — all you’d need to do to bump up the contrast of your brightfield image is to wash the cells with the dye, apply light same as before, and that specific biomolecule would be brightly lit up, distinguished from the gray mess around it.
For example, consider a chemical dye that binds to DNA. Since DNA is primarily stored in the cell’s nucleus, we can safely rely on a dye that attaches to DNA as a proxy for nucleus visualization. And, fortunately for us, there is a class of fluorescent dyes that do exactly this, often referred to as Hoechst stains.
Consider the same cell as before, but with DNA-binding dyes washed over it to the right. The nucleus lights up!
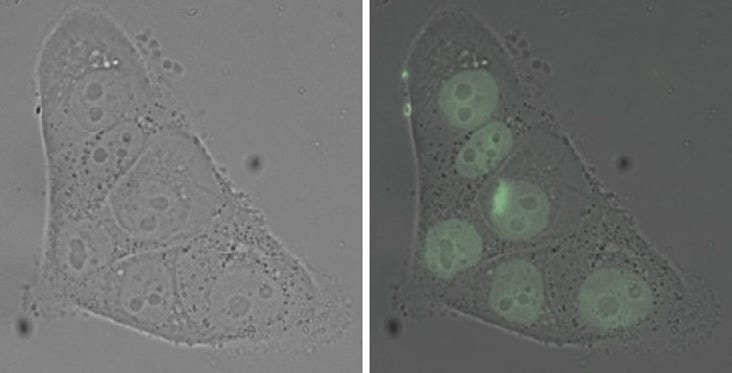
And, even more fortunately for us, there are many such dyes beyond DNA-binding ones alone. Phalloidin can bind to F-actin, revealing the cell's cytoskeleton. Wheat Germ Agglutinin can bind to sialic acid and N-acetylglucosaminyl residues, making the plasma membrane glow. And so on.
Cell painting — published in 2013 from Anne Carpenter’s lab at the Broad Institute— pushes this idea of ‘using fluorescent dyes to increase contrast’ to its logical conclusion: five to six dyes used in concert with one another, to reveal eight broadly relevant cellular components or organelles.
Quick side note: this might immediately seem like a reasonably weak ‘logical conclusion’. Why not more dyes? Why not dozens, or even hundreds? As with anything based in fluorescence, the bottleneck is in spectral overlap. You need dyes whose emission wavelengths won’t crowd each other out, and emission wavelengths are unfortunately quite large. Six simply seems to be the upper limit to ensure that you don’t face overlap.
But, you could very well correct for the overlap, which is what is often done in flow cytometry (e.g. spectral unmixing). Why don’t they do this in cell painting?
Because the assay is meant to be performed at high-throughput scales — millions of times — it is implicitly designed to meet some Pareto optimal metric. Simple to perform, contains a lot of information, and is cheap to run. This is also why the assay only uses inexpensive dyes and not, say, expensive fluorescent antibodies. Pushing it further would likely require some extra computational lifting + specialized equipment, and have questionable value. Five is simply where they landed.
And how gorgeous these dyes are!
In order, what is shown is RNA (orange), endoplasmic reticulum (green), mitochondria (red), cytoskeleton/cell-membrane (yellow), and the cell nucleus (blue). The last picture shows the overlay of all of them together.
Now that we have ultra-high-contrast images, what can we do with it?
For one, you can start to scale up the ML applied to these images. Cell painting is equivalent to a form of physical pre-processing, ensuring that the most salient parts of your microscopy image are brought into sharper focus. At the start, people quantized their cell painting images into thousands of hand-crafted features — size of cells, shape of cells, number of nuclei, and so on, using tools like CellProfiler (also created by Anne Carpenter’s lab) — and training models to predict the genetic or chemical perturbations applied to the cells. Of course, as the ML field slowly abandoned dataset priors and moved over to unstructured representations, so did the biology field. Circa 2019, using raw cell painting images as model input were confirmed as superior over hand-crafted features. This is an important point, and we’ll come back to it later.
And two, far more salient to this essay, you can start a company. Fairly, Recursion as a company was more closely aligned to general phenotypic methods for drug discovery than any one phenotyping technique. Yet, they nevertheless became closely associated with cell painting, with nearly every mention of the methodology in news articles mentioning Recursion's name and their associated grand ambitions (like so). It also doesn’t hurt that Anne Carpenter has served on their scientific advisory board since the startup was founded. From the piece, which describes Anne’s initial interactions with Recursion’s founders in 2013:
Over burgers at the nearby “Miracle of Science” pub that evening, I peppered them with questions over the course of two hours, more intensely than any PhD thesis defense I’ve witnessed. Normally, I would be a bit gentler on two grad students with a dream, but I am quite skeptical about startups by nature, and these two were planning to launch a company in the field I had pioneered: image-based profiling. I’m a bit chagrined to think how I treated them, but I certainly didn’t want a company so close to my lab’s research to hype big and fizzle out. In fact, my inherent skepticism is a large part of why I have never served on the Scientific Advisory Board of another start up company - before nor since Recursion. Survey a few MIT professors and find out how unusual that is!
But there was something special about this situation. First, I knew the science very deeply: the plan was to make use of the software my lab had created and open-sourced, called CellProfiler, to extract features from images. They would also use the image-based profiling assay my lab co-invented with Stuart Schreiber’s team, called Cell Painting, which uses cells’ morphology features extracted from images as a readout of the impact of a disease, drug, or genetic anomaly. To be fair, in 2013 I wasn’t convinced that image-based profiling would work as well as it has turned out to, and across so many applications.
And the theoretical utility of cell painting quickly bore over the years.
Models trained on raw cell painting images could predict the chemical perturbations applied to the cells. Even more interestingly, such models could even predict the mechanism of action of the perturbation — far better than models trained on purely structural chemical data alone. Their utility popped up in a variety of areas beyond that, from toxicological analysis to predicting cell stress response.
Again, this essay isn’t about whether improved phenotyping approaches (e.g. cell painting) in early R&D strongly translates to more/better actual drugs released. This hasn’t practically turned out to be the case, at least for now, though some early results suggests a reversal in that trend. As I mentioned previously, time will tell what the utility of scaling up phenotyping screens will bring.
What this essay is about is why cell painting — despite being such a seemingly promising assay — was abandoned and replaced with its century-old predecessor. To those in the ML community, the answer may be obvious: it was never needed in the first place.
The problem with cell painting
The trend of ML in general over the past 15~ years has been to strip away more and more of the biases you’ve encoded about your dataset as you feed it into a model. Computer vision went from hand-crafted interpretable features (e.g. number of circles, number of black pixels when thresholded, etc), to hand-crafted uninterpretable features (e.g. scale invariant feature transform), to automatically extracted uninterpretable features (e.g. hidden dimensions of a convolutional neural network). In other words, the bitter lesson; pre-imposing structure on your data is useful for a human, but detrimental to a machine.
Cell painting is a casualty of this truth. The method simply highlights what is already present in brightfield images, which may be useful for hand-crafted features that benefit from strong contrast, but neutral at best for deep learning methods.
As I mentioned earlier, the superiority of using raw, unaltered cell painting images as input over hand-crafted features was established by 2019, but, as time went on, the utility of cell painting at all also became suspect. Per a 2022 Scientific Reports paper, one could nearly perfectly predict what the cell painting image would be given a brightfield image.
Here is a clearer comparison from an ICCV 2023 paper, which found the same thing.
Of course, we’d be rushing if we concluded from these results alone that cell painting is useless. Perhaps there are extremely subtle, but important, differences between the ‘predicted’ and ground cell painting images that a naive eye-balling wouldn’t tell us anything about. We’d need a study that took a model trained on brightfield-only images and a model trained on cell painting-only images, and compared the two on tasks of interest.
Luckily, circa 2023, we have one of those, which compares the two modalities on predictions of chemical perturbation across ten mechanisms of action. And the results are quite clear. If you rely on CellProfiler-extracted features, cell painting wins. But, if you use raw cellular images, there is relatively little predictive difference between brightfield and cell painting images. There’s still a lot of follow-up work to do here to ensure that this trend continues across many different cells and perturbations, which is something we’ll discuss a bit more later, but it feels unlikely that this is all a coincidence.
We’ve established equivalency, at least roughly. But is there a chance that brightfield could yield something beyond cell painting alone?
Why brightfield is (maybe) even better
On face value, the value of brightfield has a lot to do with its simplicity. Cell painting is also simple, relatively, but there is a whole protocol that goes along with it. Entire consortiums have been spun up to optimize the process further, dyes cost money, and artifacts in the process may still arise. Brightfield solves all these problems. So the first-order impact of Recursion switching away from cell painting is making their dataset higher-quality, faster to acquire, and cheaper to create.
But there is another side benefit of going the brightfield route: the ability to do time-lapse microscopy. The ability to observe, in real time, how a cell behaves from second-to-second, hour-to-hour, and day-to-day.
At least some of the dyes in cell painting can be done to live cells, a few of them used in the process aren’t technically cytotoxic and were specifically chosen to minimize their effect on cell behavior. Unfortunately, behavior deviations still arise. A study from 2010 showed that the Hoechst stains — the same ones we discussed earlier that bind to DNA — can cause cell apoptosis if the dyes are repeatedly excited with light. And, in fact, the whole concept of fluorescent stains likely invariably causes levels of phototoxic effects on the cell over time.
Because of this, the cell painting assay isn’t applied to live cells! In practice, the Recursion cell painting process looks like this (following the protocol outlined here):
Grow cells.
Apply chemical compound or genetic therapy (overexpression or knockdown) or both to the cells.
Somewhere between 24-48 hours later, fix, permeabilize (allow things to pass through the cell membrane), and stain the cells with the cell painting dyes. Cell fixation refers to the process of ‘freezing’ the cells in places; preventing further cell decay by terminating ongoing biochemical reactions. The fixation chemical that Recursion relies on is paraformaldehyde, a crosslinking fixative, which forcibly creates covalent chemical bonds between the proteins in a cell and [everything] around it. At least for several weeks, this will (mostly) perfectly preserve the cell's appearance.
Within 28 days of cell dyeing + fixation, image the cells and use the resulting images for whatever you want.
There’s a reasonably strong assumption we’re making here: most of the useful information about how a perturbation affects a cell is observable at the exact moment of freezing, 24-48 hours after the perturbation first occurred. This doesn’t feel immediately obvious: there is potentially really useful data in understanding how a compound is worming its way into a cell immediately after application, whether long term changes remain on the cell morphology weeks after application, and so on. With cell painting, all that information is tossed away.
But if you use brightfield, you can image as much as you want, seeing the full temporal scope of cellular responses to perturbations.
Is there precedent to believe that viewing the temporal state of cells actually helps to understand their behavior? We certainly know that many phenotypic aspects of cells are time-bound; cell motility, the movement of membrane proteins, and so on. But do we gain anything from actually watching those time-bound events as they occur? Naively, we’d expect that to be the case. After all, look at this video of brightfield pre-adipocytes! Look how much is going on! Surely at least some of this is useful information!
(Note, I have since learned via the comments that the below video is not actually brightfield, but holotomography. The point still stands though!)
It’s…unfortunately not super clear cut from the literature.
If you try to find papers about the utility of time-lapse imaging in cells, you’ll find dozens of results, claiming that the temporal aspect is deeply important. But they are all somewhat suspect.
One paper published in Science is very outright with this, titled ‘Live-cell imaging and analysis reveal cell phenotypic transition dynamics inherently missing in snapshot data’. But all that’s actually revealed is that there is a ‘fork’ in cell state transition dynamics — not that the fork is actually meaningful in using the data for anything.
Closer to the topic of understanding the impact of chemical perturbations on cells, there is another paper titled ‘Long-term Live-cell Imaging to Assess Cell Fate in Response to Paclitaxel’. The results are a bit mixed; cell responses to chemotherapeutics do display a fair bit of heterogeneity that temporal approaches help tease out. But again, the heterogeneity is observable from the end-state, and it’s unclear how important knowing the ‘path to heterogeneity’ is.
The most relevant paper of the lot is an article titled ‘Time series modeling of live-cell shape dynamics for image-based phenotypic profiling’. Here, they more directly show that the inclusion of temporal dynamics do improve a model’s ability to separate out the phenotypes of cells treated with one drug and cells treated with another drug. But…the actual result here is incredibly weak: the ‘improvement’ in accuracy is going from correctly predicting 5 out of the 6 drugs applied to a set of cells using fixed-cell methods, to 6 out of 6 drugs. Past that, with such a small sample size of drugs/conditions, it's difficult to conclude anything from here.
Yet, despite limited evidence supporting the value of cellular dynamics, I'm going to take an unusually optimistic stance here: I strongly believe there is an immense amount of signal hidden in cell dynamics.
Most of the existing papers on the subject are extremely low sample in size, use human-interpretable features for dynamics (cell movement rates, contact time, etc), or don’t even use ML. Scaling up time-lapse microscopy and throwing sufficient-enough ML literally has never been done before. Cell dynamics is incredibly understudied, it’s very much one of those things in biology that everyone admits is probably really important, but there just was never a scalable way to study it. Until now!
There’s a really fun confluence of things going on here:
ML has gotten really, really good over the last decade, making it so extracting manual features from cell videos isn’t necessary.
Live-cell brightfield imaging can be relied upon over fixed cell painting methods.
There is a 600 person, multi-billion dollar biopharmaceutical startup that is currently collecting petabytes of time-lapse cell-perturbation brightfield videos: Recursion Pharmaceuticals.
Again, it’s an open question how useful time-lapse microscopy will be for the ultimate end goal of actually developing new drugs. Drug discovery is a graveyard of tools that sound really interesting, teach the field very cool things about biology, and ultimately end up doing nothing at all for the hard problem of making better drugs. Paying attention to the temporal dynamics of cells may very well be another entry to this graveyard: tells us a bit about some niche set of diseases, but nothing beyond that. But, regardless of what happens, I think the future here is enormously interesting.
Let’s move on. Cell painting is equivalent to brightfield and brightfield may be even better because of the potential for time-lapse microscopy. What’s Recursion to do with all this information?
The transition process
One of the immediate questions I had about the cell-painting to brightfield process wasn’t the science, but how logistically it even happened. Recursion is a platform company amongst platform companies — the cell painting assay is deeply tied to their science, their marketing, and everything about how they position themselves. Even if the science pointed towards brightfield being a good move, it would’ve been a massive undertaking for the behemoth of a startup to switch to it a decade into the game.
I talked to Charles Baker about this! He's an automation-scientist-turned-VP at Recursion Pharmaceuticals who has worked there for six years and, crucially, was deeply involved in moving the company from cell painting to brightfield imaging.
He told me that the initial inklings that brightfield was sufficient actually came from an internal 2021 hackathon project, a yearly event at Recursion dubbed ‘Hack Week’. There, a team demonstrated that brightfield images, when used as training data, gave similar results to cell painting. But what I found particularly interesting was that cell painting was still showing stronger signal than brightfield . The physical preprocessing that cell painting was doing still seemed meaningful, even if you relied on raw images as input. But there was something here, and Charles worked on exploring it further.
Biotechs often operate in a ‘don’t fix it if it isn’t broken’ mindset with regards to their primary assay (given how expensive any mistakes can be), so any attempt to modify that assay must be very de-risked. One of Charles' concerns was that brightfield may be equivalent to cell painting in some settings, but not in others. In some permutation of cell lines, perturbation, and genetic knockout, chemical dyes may suddenly become important. Because of this, papers on the subject that came from outside of the company couldn’t be outright trusted, as Recursion operated on a scale of biology that very, very few other institutions did. More testing was necessary.
And they did exactly that, across thousands of experiments. They re-adapted their software to rely on brightfield, altered sections of their lab to accommodate it, tested out many different cell lines and perturbations. Eventually, they came across a conclusion first suggested by the hackathon: it didn’t seem like there are any areas where cell painting is uniquely superior. By early 2023, Recursion had started to use brightfield imaging in their normal workflows. And, by summer 2024, brightfield imaging was Recursion’s dominant imaging modality.
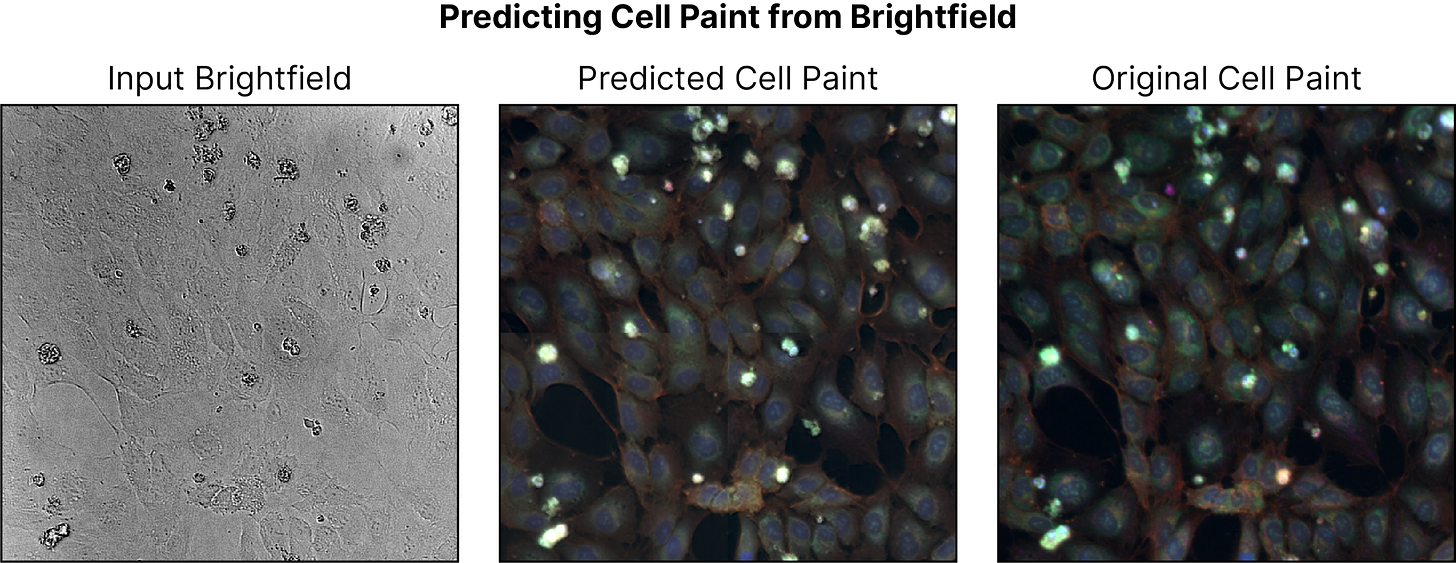
Why didn’t brightfield immediately match cell painting results in the original hackathon project? Charles had this to say: “Hackathon projects aren’t a perfect thing. We needed more time spent training the model. We also benefited from capturing data in additional cell types to increase the diversity of the training data and allow the model to generalize. That data collection happened after Hack Week.".
How hard was this? Surprisingly, pretty simple in Charles' eyes. People were generally excited once the (very high) bar for equivalency had been met — it helps that brightfield is way nicer to run at scale than cell painting — so the whole transition process was a fair bit less painful than I had initially assumed it’d be.
What happened to the millions of images and petabytes of cell painting data that Recursion had collected over the last decade? I asked Charles about this, and he said there are no plans to deprecate any of it. After all, it’s all really the same data, represented with a visually different modality, but information-wise the same. He did suggest the possibility that cell painting may still be relied upon in some cases. The two years of brightfield-testing that Recursion did couldn’t possibly cover every edge case, and there’s always the chance that there are some cell lines or perturbations that are insufficiently captured via brightfield. So their models will be taught with both modalities for now.
Finally, I asked about something I’ve been harping on in this essay: is there much utility in the time-lapse microscopy unlocked via brightfield? Unfortunately, the answer is still hazy. Charles agreed that dynamics is understudied, that there’s a lot of new biology there, and that he’s excited about exploring it. But how it impacts drug discovery is still something to be determined. After all, the lag time for the value-add of these sorts of things are always incredibly long. For now, Recursion is imaging on a day-by-day level, which is more relevant for genetic perturbations than for chemical ones (where second-by-second imaging is more useful), and testing out how useful those coarse-grained videos are. He also said that even if dynamics turns out to not be useful, the move to brightfield imaging is still saving an enormous amount of money, time, and man-hours, so it’s still worth it.
And…that wraps up this essay! It ended up being much longer than expected, but happy that I covered the subject in the detail I wanted. I’m deeply interested in writing more of these sorts of ‘untold scientific stories’ and deeply expanding on the scientific nuances of them, so please reach out to me if you have one that you’d want told! And again, shout-out to Brita Belli and Charles Baker for talking to me and editing the final draft of this piece!
Brita noted that the internal results of the Phase 2 trial were treated pretty positively internally at Recursion, given positive safety results and positive secondary endpoint results. How positive were these secondary endpoints? Some context: CCM, the disease the phase 2 trial drug was for, stands for Cerebral Cavernous Malformation, which is a ‘a rare condition that occurs when a collection of small blood vessels in the brain or spinal cord become enlarged, irregular, and prone to leaking’. From the press release, the results of the trial were:
Magnetic resonance imaging-based secondary efficacy endpoints showed a trend towards reduced lesion volume and hemosiderin ring size in patients at the highest dose (400mg) as compared to placebo. Time-dependent improvement in these trends at the 400mg dose was also observed in this signal-finding study. Improvements in either patient or physician-reported outcomes were not yet seen at the 12 month time point.
How much should we trust the utility of these secondary endpoints?
Lesion volume didn’t seem to be related to outcomes (outside of severe cases) in one study in children. The primary issue with CCM lesions seems to not be their size, but their permeability, which doesn’t correlate well with size. Lesion sizes also seem to be dynamic, potentially shrinking while disease progression gets worse (though, in the long term, the lesion always gets larger).
Removal of the hemosiderin ring does seem to sometimes be beneficial, but it does seem to be somewhat controversial how useful it is. I’m unsure how well reduction of the size of ring maps onto surgical removal of the ring, but it’s the only parallel we can draw here given that CCM treatments are generally all surgical.
Because of this all, I’d be bearish on this particular drug, unless the time-dependent improvements really pull through. It’s also possible there is something interesting in the data that has yet to be released; why else would Recursion pay for another trial? Again though, this isn’t hating on Recursion’s platform. REC-994 is barely a reflection of the power of phenomics (see the footnote below this).
CEO’s PhD project’ phrase comes up really often when referring to this first REC-994 drug, but nobody ever seems to expand on it. I finally found an article that discussed it more deeply, here are the important excerpts glued together:
Gibson hit on the opportunity offered by machine learning in cell imaging when he was a PhD student in Dean Li’s lab, at the University of Utah, unravelling the biology of cerebral cavernous malformation (CCM)…
Frustrated by the pitfalls of the target-first approach to drug discovery, the team developed a phenotypic screen to hunt for its next set of leads…
Gibson, faced with the prospect of having to manually review the images of cells to identify hits, set up a machine learning programme to cluster the drugs on the basis of their overall morphological effects…
Two computer-suggested candidates — tempol and cholecalciferol — passed with flying colours in secondary, tertiary and quaternary follow-up screens…
Their phase I candidate REC-994 [now Phase 2] is the superoxide dismutase mimetic tempol, one of the compounds that Gibson’s algorithm initially identified as a candidate for treating CCM.
TLDR: REC-994 is genuinely the result of a PhD project and is a bad reflection of how good Recursion-produced drugs could be.
This is your strongest post yet. Really interesting and well-explained about a subject that I'm not that familiar with.
Perfect timing for me! Thank you for that case study I hadn't seen! (https://doi.org/10.1371/journal.pcbi.1011323)
The other two cases were only 3 stains, but this one used all 5 seen in CellPaint. If brightfield can really extract this much information using strictly ML methods it means it should be able to extract information about other molecular locations in the cell that are not normally stained, making it better than CellPaint for reasons other than fixation/spectrum limitations.